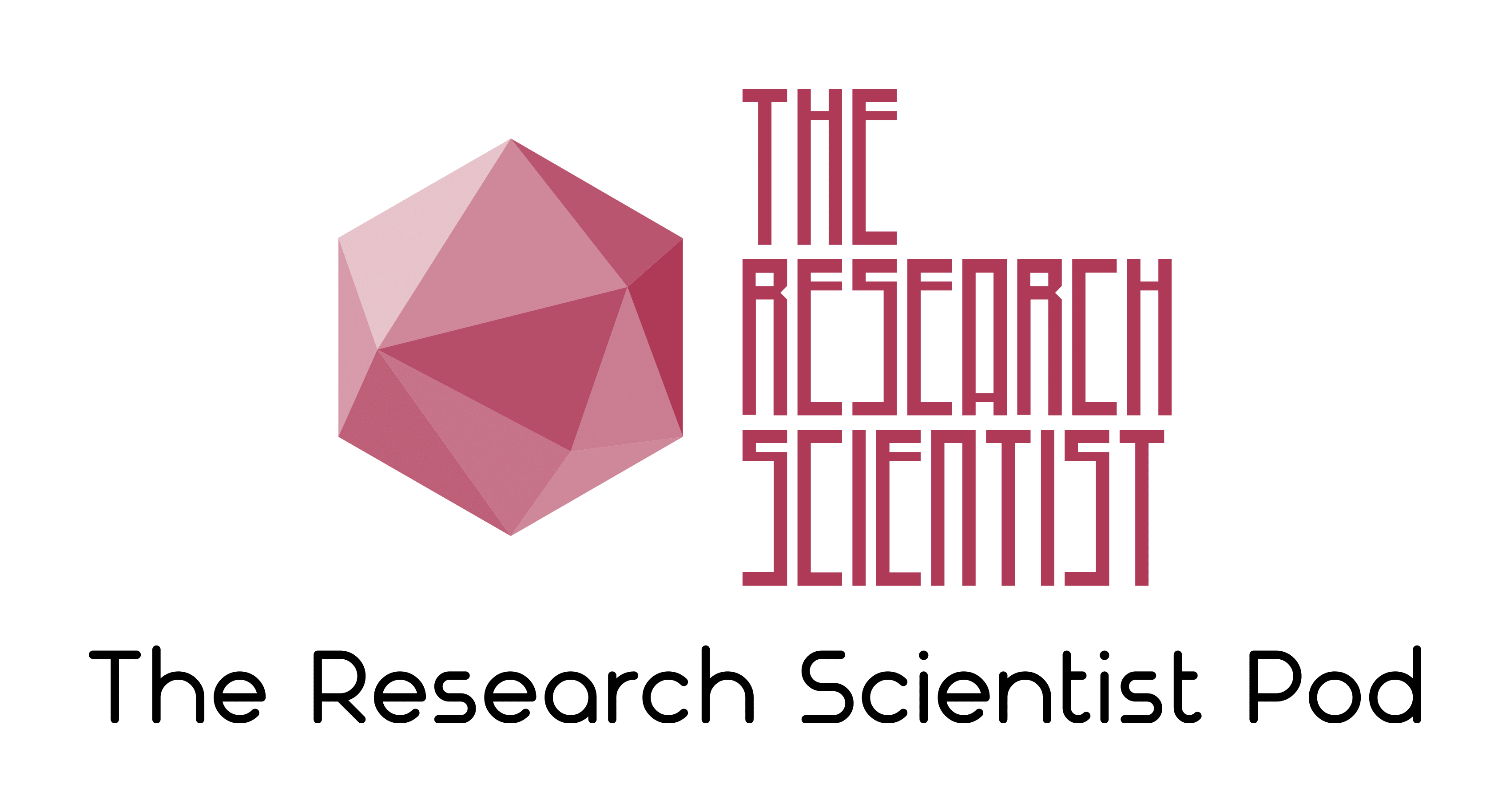
Calculate the Mean Squared Error between predicted and observed values. Input your values and visualize the differences between them.
Mean Squared Error (MSE):
Understanding Mean Squared Error (MSE)
The Mean Squared Error (MSE) measures the average squared difference between predicted values (Pi) and observed values (Oi). It is widely used to assess the performance of regression models.
Formula for Mean Squared Error
Example Calculation
Suppose we have the following predicted and observed values:
- Predicted values: [2.3, 3.1, 4.0]
- Observed values: [2.5, 3.0, 3.8]
The MSE is calculated as follows:
Step 1: Compute the differences between each pair of predicted and observed values:
- Difference 1: \( 2.3 - 2.5 = -0.2 \)
- Difference 2: \( 3.1 - 3.0 = 0.1 \)
- Difference 3: \( 4.0 - 3.8 = 0.2 \)
Step 2: Square each of the differences:
- \((-0.2)^2 = 0.04\)
- \((0.1)^2 = 0.01\)
- \((0.2)^2 = 0.04\)
Step 3: Sum the squared differences:
- \( 0.04 + 0.01 + 0.04 = 0.09 \)
Step 4: Divide by the number of data points (\(n = 3\)):
- \( \text{MSE} = \frac{0.09}{3} = 0.03 \)
Thus, the Mean Squared Error (MSE) for this example is: 0.03
Further Reading
Implementations
Attribution
If you found this guide helpful, feel free to link back to this post for attribution and share it with others!