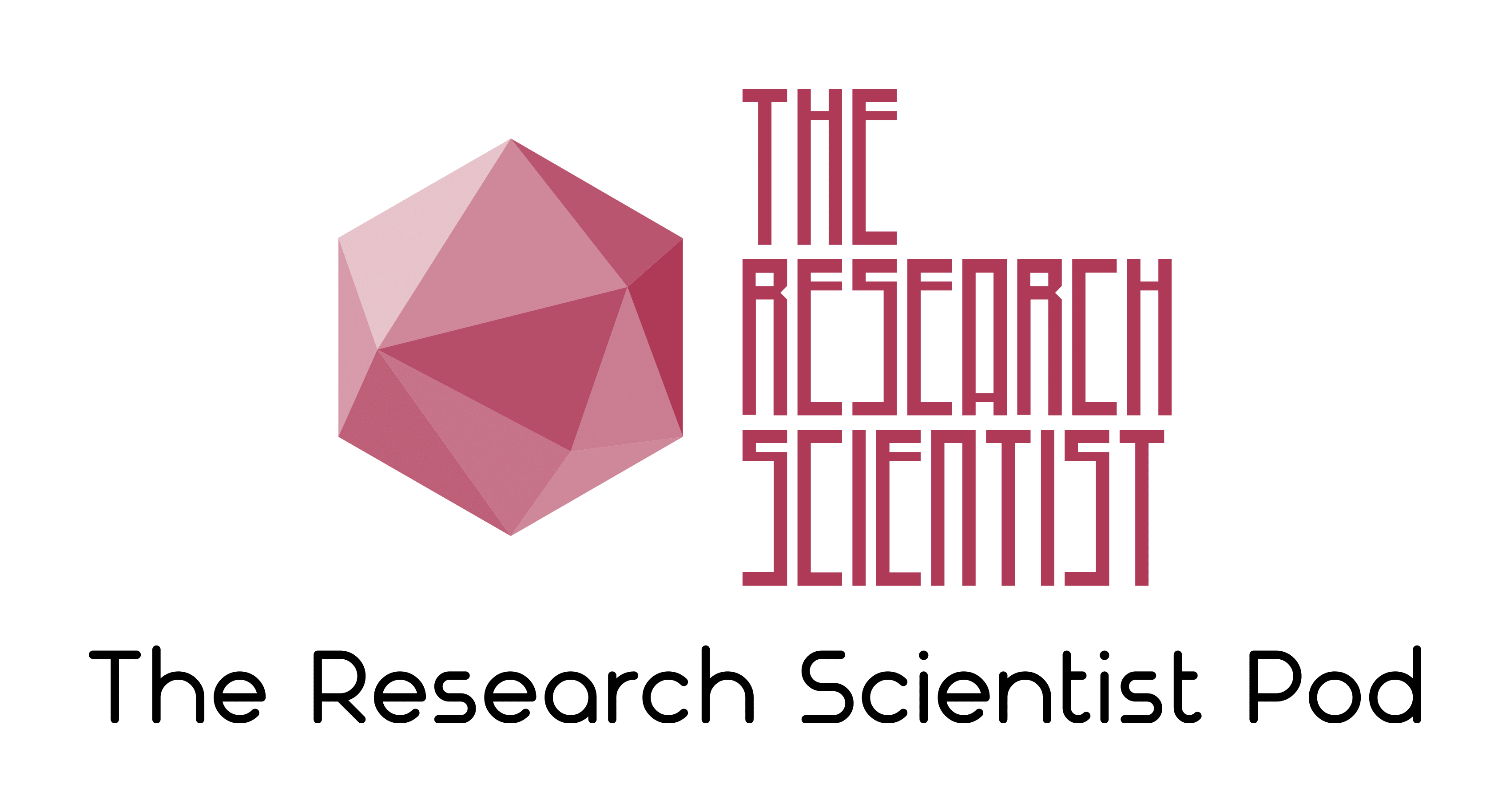
Use this calculator to compute probabilities, percentiles, and visualize the normal distribution. Input the population mean, standard deviation, and the specific point(s) to calculate left, right, between, or outside probabilities.
Calculated Probability:
Understanding the Normal Distribution
The normal distribution, also known as the Gaussian distribution, is one of the most important probability distributions in statistics. It describes how the values of a variable are distributed ā most values cluster around a central region, and values become less frequent as they move further from the center.
Key Characteristics of the Normal Distribution
- Symmetry: The normal distribution is perfectly symmetrical around its mean. This means that the left and right halves of the distribution are mirror images.
- Bell-shaped Curve: The distribution forms a bell shape, with the peak at the mean (μ) and tails extending indefinitely in both directions.
- Mean, Median, Mode: In a normal distribution, the mean, median, and mode are equal and all located at the center of the distribution.
- 68-95-99.7 Rule: Also known as the empirical rule, about 68% of data falls within one standard deviation (Ļ) of the mean, 95% within two, and 99.7% within three standard deviations.
Conditions for Using the Normal Distribution
While the normal distribution applies to many real-world scenarios, it is important to check the following conditions:
- Independence: The data points should be independent of each other. One data point should not influence the next.
- Sample Size: When applying the Central Limit Theorem (CLT), ensure your sample size is sufficiently large (generally, n ā„ 30) to assume a normal distribution of sample means, regardless of the population distribution.
- No Extreme Skewness: The normal distribution is symmetric. If your data has extreme skewness or outliers, it may not fit a normal distribution well, even with a large sample size.
Caveats and Assumptions
- Small sample sizes or data with significant skewness may require a transformation or alternative distributions (e.g., t-distribution) for accurate analysis.
- The Central Limit Theorem (CLT) applies to sample means, meaning that with a large enough sample size, the distribution of the sample means will approximate a normal distribution, even if the population distribution is not normal.
- Assuming normality is a common approach in many statistical models, but it is always important to check the fit before making inferences.