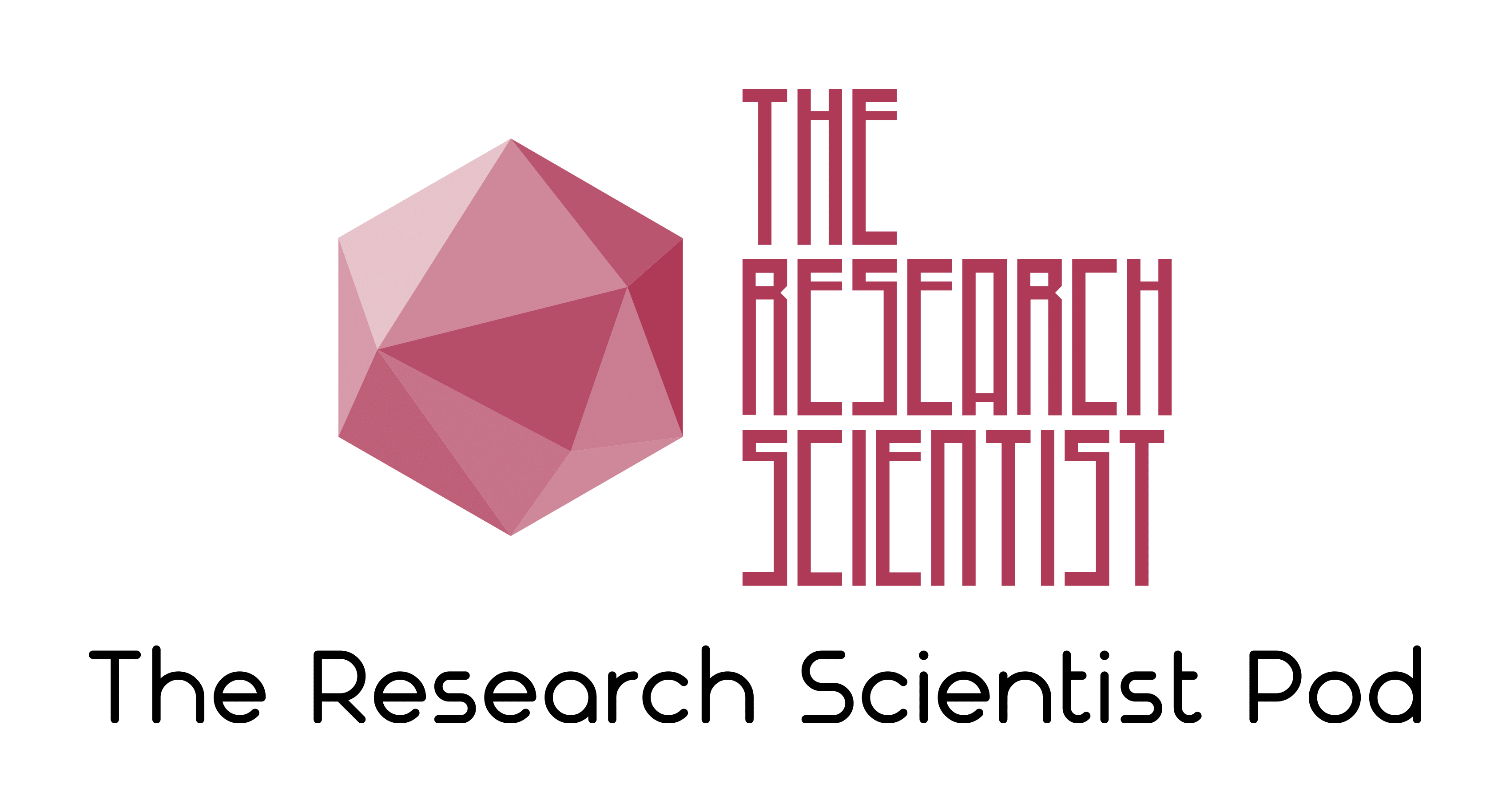
Enter the number of successes and sample size to calculate different point estimates for a population proportion.
Maximum Likelihood Estimate (MLE):
Wilson Estimate:
Jeffreys Estimate:
Laplace Estimate:
Understanding Different Point Estimates for Proportion
1. Maximum Likelihood Estimate (MLE)
The MLE is the ratio of observed successes to the total sample size:
2. Wilson Estimate
The Wilson Estimate adjusts for small sample sizes and is calculated as:
where \( Z \) is the Z-score for the selected confidence level.
3. Jeffreys Estimate
The Jeffreys Estimate is a Bayesian estimator that assumes a prior of Beta(0.5, 0.5):
4. Laplace Estimate
The Laplace Estimate also uses a Bayesian approach, with a prior of Beta(1, 1):
Real-Life Example: Customer Satisfaction Survey
Suppose a company conducts a survey among 50 customers to determine the proportion of satisfied customers. Out of these 50 customers, 35 report being satisfied.
- Step 1: The observed number of successes \( x = 35 \) and sample size \( n = 50 \).
- Step 2: Calculate each estimate:
- MLE: \( \hat{p}_{MLE} = \frac{35}{50} = 0.70 \)
- Wilson Estimate: Using \( Z = 1.96 \) for 95% confidence, \[ \hat{p}_{Wilson} = \frac{35 + \frac{1.96^2}{2}}{50 + 1.96^2} \approx 0.701 \]
- Jeffreys Estimate: \[ \hat{p}_{Jeffreys} = \frac{35 + 0.5}{50 + 1} = 0.695 \]
- Laplace Estimate: \[ \hat{p}_{Laplace} = \frac{35 + 1}{50 + 2} = 0.692 \]
- Interpretation: The company can use these different estimates to better understand the true proportion of satisfied customers. Each estimate provides slightly different values based on assumptions and adjustments for sample size.