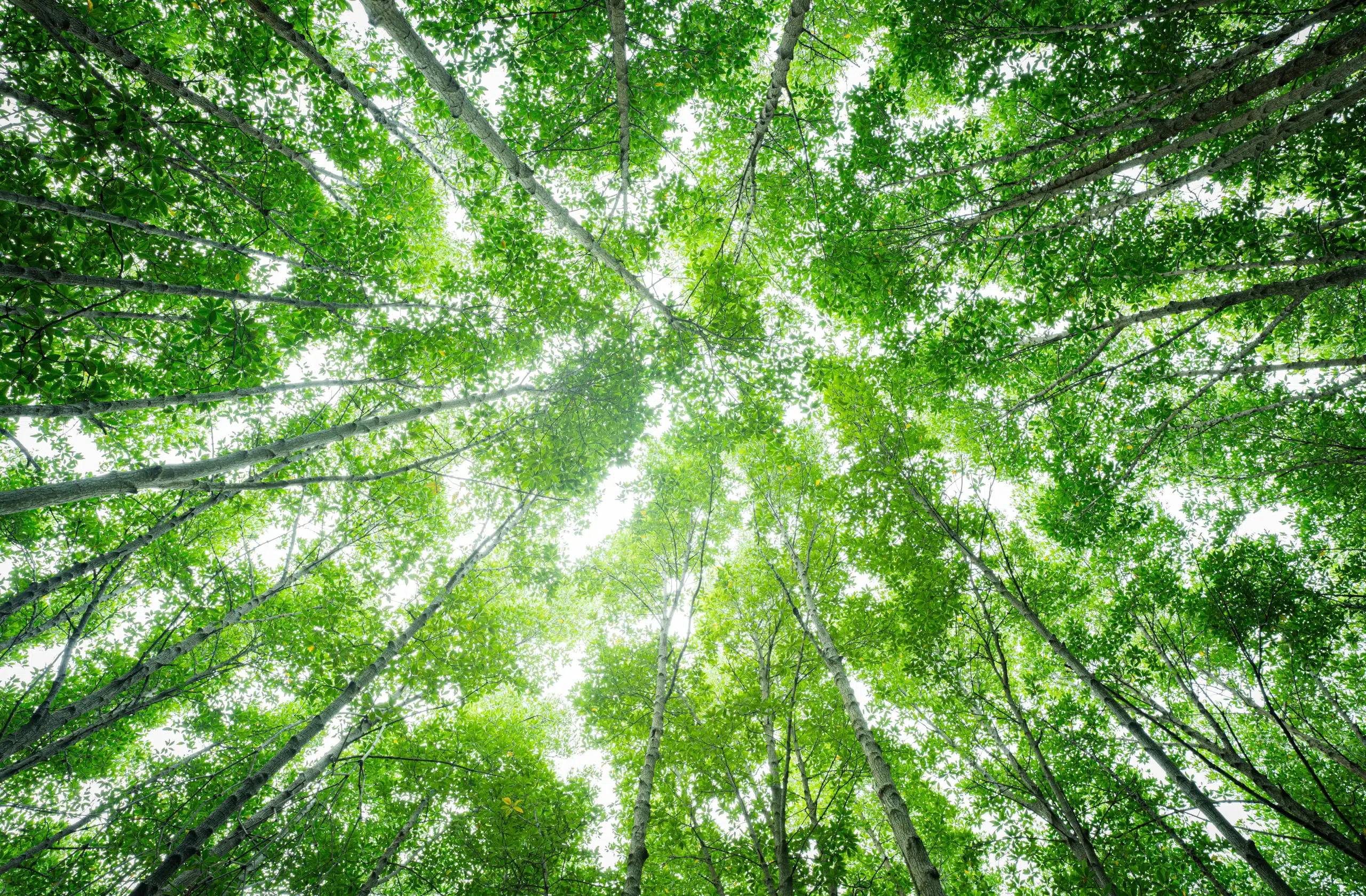
In the realm of ecological research, understanding and quantifying biodiversity is crucial for conservation efforts, ecosystem management, and tracking environmental change. Two fundamental tools that ecologists use to measure biodiversity are the Shannon Diversity Index (also known as the Shannon-Wiener Index, Shannon-Weaver Index, or Shannon Entropy) and its companion metric, Equitability. These mathematical tools help us understand not just how many species are present in an ecosystem, but also how evenly distributed they are—a crucial distinction that can reveal important patterns in ecosystem health and stability.
Note: While the name “Shannon-Weaver Index” is commonly used, it’s worth noting that the diversity index was developed solely by Claude Shannon. Warren Weaver was involved in the publication where Shannon first introduced his information theory, but not in developing the diversity index itself. Some ecologists prefer using “Shannon Index” or “Shannon-Wiener Index” to reflect this historical accuracy.
Contents
- The Foundation: Shannon’s Information Theory
- Practical Applications Through Examples
- Ecological Significance and Interpretation
- Interpreting and Applying the Indices
- Practical Considerations in Application
- Alternative Biodiversity Metrics
- Programmatic Implementation
- Conclusion
- Further Reading
- Attribution and Citation
The Foundation: Shannon’s Information Theory
The Shannon Diversity Index, originally developed by Claude Shannon for information theory, has found a perfect application in ecology. Just as Shannon used his formula to measure the information content in messages, ecologists use it to measure the “information content” in an ecosystem’s species distribution. The more species present and the more evenly they are distributed, the higher the “information content” or diversity of the system.
Understanding the Mathematics
The Shannon Diversity Index (H) is expressed mathematically as:
\[ H = -\sum_{i=1}^{s} p_i \ln(p_i) \]In this equation, each component serves a specific purpose:
- \(p_i\) represents the proportion of individuals belonging to species i in the dataset (where i is simply a counter for each species)
- \(s\) stands for the total number of species found in the community (species richness)
- \(\ln\) is the natural logarithm (base e ≈ 2.718), chosen instead of the base-10 logarithm because it provides better mathematical properties for measuring diversity. Natural logarithms are particularly useful in ecological studies as they better reflect the continuous nature of biological processes.
Building on this, Equitability (J) provides a way to standardize the diversity measure:
\[ J = \frac{H}{H_{max}} = \frac{H}{\ln(s)} \]This standardization allows us to compare communities with different numbers of species by expressing how close the observed diversity is to the maximum possible diversity for that number of species.
Practical Applications Through Examples
Example 1: Forest Understory Diversity
Let’s explore a real-world example by analyzing the diversity of understory plants in a forest plot. This example will demonstrate how to calculate both indices and interpret their ecological significance.
Species | Number of Individuals | Proportion (p₁, p₂, etc.) | p × ln(p) |
---|---|---|---|
Wood Fern | 30 | 0.300 | -0.361 |
Wild Ginger | 25 | 0.250 | -0.347 |
Trillium | 20 | 0.200 | -0.322 |
Jack-in-the-pulpit | 15 | 0.150 | -0.284 |
Wild Violet | 10 | 0.100 | -0.230 |
Let’s walk through the calculation process step by step:
First, we calculate the proportion of each species by dividing its count by the total number of individuals (100 in this case). For example, Wood Fern has 30 individuals, so its proportion is 30/100 = 0.300.
Next, for each species, we multiply its proportion by the natural logarithm of that proportion. For Wood Fern, this is:
0.300 × ln(0.300) = 0.300 × (-1.204) = -0.361
We sum all these products and take the negative of the sum. Let’s show this step explicitly:
H = -(-0.361 + -0.347 + -0.322 + -0.284 + -0.230)
H = -(−1.544)
H = 1.544
To calculate Equitability, we need the maximum possible diversity for five species:
H_max = ln(5) = 1.609
Therefore, our Equitability is:
J = 1.544/1.609 = 0.960
Example 2: Comparing Disturbed and Undisturbed Habitats
To understand how these indices can reveal ecosystem changes, let’s compare two sites with identical species counts but different distributions—a situation often encountered when studying habitat disturbance.
Undisturbed Forest Site
Species | Count | Proportion |
---|---|---|
Oak Seedlings | 20 | 0.333 |
Maple Seedlings | 20 | 0.333 |
Beech Seedlings | 20 | 0.333 |
Disturbed Forest Site
Species | Count | Proportion |
---|---|---|
Oak Seedlings | 50 | 0.833 |
Maple Seedlings | 5 | 0.083 |
Beech Seedlings | 5 | 0.083 |
The calculations reveal striking differences:
For the undisturbed site:
H = -3(0.333 × ln(0.333)) = 1.099
J = 1.099/ln(3) = 1.000
For the disturbed site:
H = -(0.833 × ln(0.833) + 2(0.083 × ln(0.083))) = 0.451
J = 0.451/ln(3) = 0.410
These results tell a clear story: while both sites have the same number of species, the disturbed site shows dramatically lower diversity and evenness. This pattern is typical of disturbed environments where stress-tolerant species often dominate while others struggle to maintain their populations.
Ecological Significance and Interpretation
The values we calculate through these indices have profound implications for ecosystem function and stability. A high Equitability value (like our forest example’s 0.960) indicates more than just mathematical evenness—it suggests several important ecological characteristics:
Ecological Implications of High Equitability
When a community shows high equitability (J approaching 1.000), it often indicates:
Resource Partitioning: Species have evolved to utilize different ecological niches, reducing direct competition and allowing stable coexistence. In our forest understory example, the even distribution suggests each species has carved out its own specific role in the ecosystem, whether through different rooting depths, light requirements, or nutrient needs.
Ecosystem Resilience: Communities with high equitability often show greater resistance to disturbance and faster recovery after environmental stress. If one species declines, others can often compensate by expanding their ecological roles, maintaining overall ecosystem function.
Balanced Trophic Interactions: Even species distribution often supports more stable food webs and pollination networks. In our understory example, this might mean more reliable resources for various herbivores and pollinators throughout the growing season.
Evolutionary History: High equitability often reflects a long history of coevolution and community assembly, where species have developed complementary rather than competitive relationships.
Interpreting Low Equitability
Conversely, when we observe low equitability (as in our disturbed forest site with J = 0.410), it often signals ecological stress or recent disturbance. The dominance of one species (like the oak seedlings in our example) might indicate:
• Environmental filtering where only certain species can tolerate current conditions
• Recent disturbance that has disrupted normal competitive relationships
• Potential ecosystem instability and reduced resilience to future changes
• Simplified trophic interactions that might affect ecosystem services
Interpreting and Applying the Indices
When working with the Shannon Diversity Index and Equitability, several key principles guide our interpretation. The Shannon Index typically ranges from 1.5 to 3.5 in most ecological studies, with higher values indicating greater diversity. However, the actual value is less important than comparisons between similar ecosystems or changes within the same ecosystem over time.
Equitability, ranging from 0 to 1, provides a more standardized measure. A value near 1 indicates species are present in nearly equal numbers, while values closer to 0 suggest dominance by one or a few species. This metric is particularly valuable for comparing communities with different numbers of species, as it accounts for the maximum possible diversity given the number of species present.
Practical Considerations in Application
When applying these indices in ecological research, several practical considerations deserve attention. Sample size significantly affects our ability to detect and accurately represent rare species, which in turn influences our diversity calculations. It’s essential to ensure sampling effort is consistent when comparing different sites or time periods.
Additionally, these indices should be used as part of a broader suite of biodiversity metrics. While they provide valuable insights into community structure, they don’t capture all aspects of biodiversity. For instance, they don’t account for species’ functional roles or evolutionary relationships, which might be crucial for conservation planning.
Practical Tips for Field Application
When conducting biodiversity surveys and applying these indices, consider the following guidelines:
First, ensure your sampling area is appropriate for the organism type and habitat being studied. Different ecosystems and organisms require different sampling approaches to obtain representative data.
Second, maintain consistent sampling effort across sites and time periods. Variations in sampling effort can create artificial differences in diversity measurements that don’t reflect real ecological patterns.
Third, document your sampling methodology thoroughly, including any limitations or potential biases. This information is crucial for interpreting results and comparing them with other studies.
Finally, consider seasonal variations in species presence and abundance. Multiple sampling periods throughout the year might be necessary to capture the full diversity of some communities.
Alternative Biodiversity Metrics
While the Shannon Diversity Index is widely used, it’s important to recognize it as one of several valuable tools for measuring biodiversity. Each metric offers unique insights:
Simpson’s Diversity Index (D) – Particularly sensitive to dominant species, making it useful when abundant species are of primary interest. Less affected by rare species than Shannon’s Index. Calculated as:
\[ D = 1 – \sum_{i=1}^{s} p_i^2 \]Species Richness (S) – The simplest measure, counting only the number of different species present. Useful for quick assessments but doesn’t account for relative abundances.
Hill Numbers – A unified framework that includes both Shannon and Simpson indices as special cases, allowing for consistent comparison across different diversity orders.
For comprehensive biodiversity assessment, consider using multiple indices together:
- Shannon Index when evenness and rare species are important
- Simpson’s Index when focusing on dominant species
- Species Richness for basic diversity assessment
- Hill Numbers when comparing across different ecological scales
Programmatic Implementation
Let’s explore how to calculate the Shannon Diversity Index and Equitability using both Python and R. We’ll implement functions that can handle any species abundance data and demonstrate their use with our forest understory example.
Python Implementation
import numpy as np
import pandas as pd
def shannon_diversity(abundances):
"""
Calculate Shannon Diversity Index and Equitability from species abundances.
Parameters:
-----------
abundances : array-like
List or array of species abundances (counts)
Returns:
--------
tuple : (float, float)
Shannon Diversity Index (H) and Equitability (J)
"""
# Convert to numpy array and ensure positive values
abundances = np.array(abundances)
abundances = abundances[abundances > 0]
# Calculate proportions and total abundance
total = abundances.sum()
proportions = abundances / total
# Calculate Shannon Index
H = -np.sum(proportions * np.log(proportions))
# Calculate maximum possible diversity
H_max = np.log(len(abundances))
# Calculate Equitability
J = H / H_max
return H, J
# Example usage with our forest understory data
species_counts = [30, 25, 20, 15, 10] # Wood Fern, Wild Ginger, etc.
species_names = ['Wood Fern', 'Wild Ginger', 'Trillium',
'Jack-in-the-pulpit', 'Wild Violet']
# Create a pandas DataFrame for better visualization
df = pd.DataFrame({
'Species': species_names,
'Count': species_counts
})
# Calculate diversity indices
H, J = shannon_diversity(species_counts)
print(f"Shannon Diversity Index (H): {H:.3f}")
print(f"Equitability (J): {J:.3f}")
Shannon Diversity Index (H): 1.544
Equitability (J): 0.960
R Implementation
# Function to calculate Shannon Diversity Index and Equitability
shannon_diversity <- function(abundances) {
# Remove zeros and convert to numeric
abundances <- as.numeric(abundances[abundances > 0])
# Calculate total abundance and proportions
total <- sum(abundances)
proportions <- abundances / total
# Calculate Shannon Index
H <- -sum(proportions * log(proportions))
# Calculate maximum possible diversity
H_max <- log(length(abundances))
# Calculate Equitability
J <- H / H_max
# Return both metrics
return(list(shannon_index = H, equitability = J))
}
# Example usage with our forest understory data
species_counts <- c(30, 25, 20, 15, 10) # Wood Fern, Wild Ginger, etc.
species_names <- c('Wood Fern', 'Wild Ginger', 'Trillium',
'Jack-in-the-pulpit', 'Wild Violet')
# Create a data frame for better organization
df <- data.frame(
Species = species_names,
Count = species_counts
)
# Calculate diversity indices
results <- shannon_diversity(df$Count)
# Print results
cat(sprintf("Shannon Diversity Index (H): %.3f\n", results$shannon_index))
cat(sprintf("Equitability (J): %.3f\n", results$equitability))
# Using vegan package (alternative approach)
# Install package if required
install.packages('vegan')
library(vegan)
# Calculate Shannon diversity using vegan
H_vegan <- diversity(species_counts, index = "shannon")
# Calculate Equitability using vegan
J_vegan <- H_vegan / log(length(species_counts))
cat("\nUsing vegan package:\n")
cat(sprintf("Shannon Diversity Index (H): %.3f\n", H_vegan))
cat(sprintf("Equitability (J): %.3f\n", J_vegan))
Shannon Diversity Index (H): 1.544
Equitability (J): 0.960
Using vegan package:
Shannon Diversity Index (H): 1.544
Equitability (J): 0.960
Implementation Notes
Python Implementation:
- Uses NumPy for efficient numerical computations
- Includes data validation and handling of zero abundances
- Returns both indices as a tuple for easy unpacking
- Optional Pandas integration for data organization
R Implementation:
- Provides both base R and vegan package implementations.
- Handles data validation similarly to Python version
- Returns results in a list for flexibility
- Demonstrates consistency between custom function and established package
The vegan package is a comprehensive R package for community ecologists, offering tools for diversity analysis, species abundance modeling, and ordination methods. It's considered the standard package for biodiversity computations in R, providing functions for calculating not just Shannon diversity but also other indices like Simpson's, Chao, and rarefaction curves.
Conclusion
The Shannon Diversity Index and Equitability are powerful tools for understanding community structure and ecosystem health. By providing quantitative measures of both species richness and evenness, they enable ecologists to track changes in biodiversity, assess the impacts of environmental disturbance, and guide conservation efforts. While these metrics have their limitations, their consistent application and careful interpretation continue to provide valuable insights into the complexity and dynamics of ecological communities.
Further Reading
-
Shannon Diversity Calculator
Our dedicated calculator helps you compute Shannon Diversity Index and Equitability quickly and accurately, complete with step-by-step explanations and visualizations of the calculation process.
-
Simpson Diversity Calculator
Explore an alternative measure of diversity with our Simpson Index calculator, which emphasizes dominant species differently than the Shannon Index.
-
Sørensen-Dice Coefficient: A Comprehensive Guide
Master similarity measurement with the Sørensen-Dice coefficient, including implementations in Python and R, applications in medical imaging, text analysis, and ecological research.
-
Bray-Curtis Dissimilarity: A Comprehensive Guide
Master ecological distance measurement with Bray-Curtis dissimilarity, including Python and R implementations, applications in community ecology, biodiversity assessment, and environmental monitoring.
-
Shannon diversity index: a call to replace the original Shannon’s formula with unbiased estimator in the population genetics studies.
This paper investigates the impact of sample size and locus properties on the accuracy of different estimators of Shannon's diversity index in population genetics studies, comparing the original Shannon index with unbiased estimators proposed by Zahl, Chao & Shen, and Chao et al., and recommends replacing the original Shannon index with Zahl's unbiased estimator.
-
A conceptual guide to measuring species diversity
This paper advocates for using coverage-based sampling and Hill diversity metrics to obtain more robust and comparable estimates of species diversity in ecological studies, addressing the limitations of traditional methods like species richness, Shannon index, and Simpson index.
Attribution and Citation
If you found this guide and tools helpful, feel free to link back to this page or cite it in your work!
Suf is a senior advisor in data science with deep expertise in Natural Language Processing, Complex Networks, and Anomaly Detection. Formerly a postdoctoral research fellow, he applied advanced physics techniques to tackle real-world, data-heavy industry challenges. Before that, he was a particle physicist at the ATLAS Experiment of the Large Hadron Collider. Now, he’s focused on bringing more fun and curiosity to the world of science and research online.